Lennart Heim is the Compute Team Lead at RAND’s Technology and Security Policy Center and a Professor of Policy Analysis at RAND School of Public Policy.
China will likely match U.S. AI model capabilities this year, triggering inevitable concerns about America’s technological edge. However, this snapshot comparison misses the bigger picture. While Chinese models close the gap on benchmarks, the U.S. maintains an advantage in total compute capacity — owning far more, and more advanced, AI chips. This compute advantage, if leveraged strategically, will play an extraordinary role in driving economic transformation, securing technological leadership, and shaping the global AI ecosystem. U.S. policymakers risk squandering this edge by focusing on the wrong metrics and overreacting to predictable Chinese advancements.
TSMC's Secret Pipeline to China
Central to the U.S.-China AI competition are U.S. export controls that restrict China from importing advanced AI chips, acquiring semiconductor manufacturing equipment to build indigenous advanced AI chips, and using leading chip manufacturers such as Taiwan Semiconductor Manufacturing Company (TSMC). Despite these measures, a massive failure occurred in September 2024: TSMC, lacking basic due diligence, breached export restrictions by producing advanced AI chips for Huawei through a Chinese proxy company. This violation allowed Huawei to secure approximately 3 million chip dies using TSMC’s 7nm process, enabling the production of China’s best AI chips, the Huawei Ascend 910B and the upcoming 910C. This dwarfs even the highest estimates of smuggled chips, which typically involved tens of thousands of units, not millions. Although these chips trail the U.S. state of the art by about four years, they collectively provide China with computing power equivalent to approximately 1 million export-controlled Nvidia H100s (Nvidia's previous-generation chip from 2023) — substantial AI compute capacity that compensates for China's lack of indigenous production capabilities.
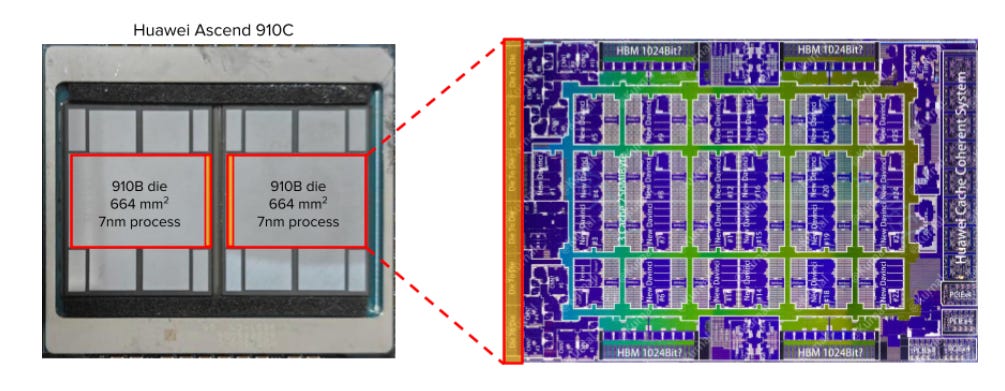
A Pattern of Failures, Not an Exception
The U.S. government responded to TSMC’s illicit production issue with a harsher rule in January 2025 and a likely investigation, but this incident is just one of many failures.
The 2022 chip controls contained specification errors that allowed Nvidia to slightly modify existing chips, creating the A800 and H800, which enabled DeepSeek's rise. Entity-listed Chinese companies built literal bridges to access advanced chipmaking equipment in unlisted facilities. Chinese firms stockpiled enough high-bandwidth memory (HBM) to meet their needs for the next few years, after the industry leaked the December 2024 restrictions in July. Until recently, AI chips with deployment-optimized performance, such as Nvidia's H20, continued to flow to China despite evidence that they enable new reasoning capabilities, with U.S. government officials taking months to act despite warnings. In every case: too little, too late.
What to Expect from Export Controls—And What Not to Expect
Despite these implementation failures, export controls remain a valuable strategic tool. While export controls do not create absolute barriers, they impose costs that have substantially slowed China's progress in AI and semiconductors, thereby maintaining America's lead.
But why is China still producing competitive models? It's harder for export controls to affect individual training runs than an entire ecosystem. Think of AI compute like factory equipment: Having fewer production lines doesn't prevent you from manufacturing a single product—you might still create that flagship product—but it severely limits your production capacity and market reach. Without the latest machinery, your production costs are higher and efficiency lower while competitors optimize their operations. What's more, the economics of scale work against you: while your competitor with ten factories can spread fixed costs across massive production volumes, driving down unit costs and enabling experimentation with new product lines, you're stuck with higher per-unit costs and limited ability to diversify. Similarly, when China has less total compute, they can still develop competitive models. After all, even in the U.S., companies only spent a fraction of their compute on training frontier models. But China misses out on the economies of scale that allow the U.S. to deploy AI broadly, experiment with more approaches, support a diverse ecosystem of leading AI companies, and continuously reinvest efficiency gains across their entire AI economy. Critically, this compute disadvantage also limits China's ability to project soft power globally—whether by providing AI services to international markets or by having the capacity to export advanced AI chips to other nations.
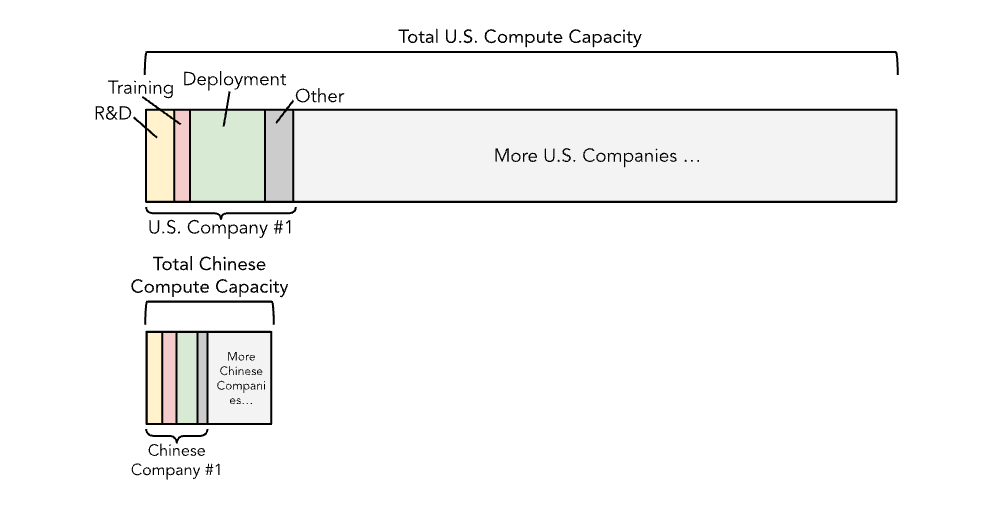
However, if current trends in AI training continue—shifting from two chips in 2012 to clusters of hundreds of thousands in 2025—export controls may eventually bite harder. China would need to build significantly larger clusters to compensate for less powerful chips: a cluster of 100,000 Nvidia B200s (a leading U.S. AI chip) might require a Chinese equivalent of 300,000 Ascend 910Cs (China's leading chip), resulting in higher energy consumption and greater engineering complexity for distributing AI workloads. Compounding this hardware gap is Nvidia's sophisticated software and networking ecosystem—crucial for orchestrating massive AI chip clusters and currently unmatched by Chinese alternatives.
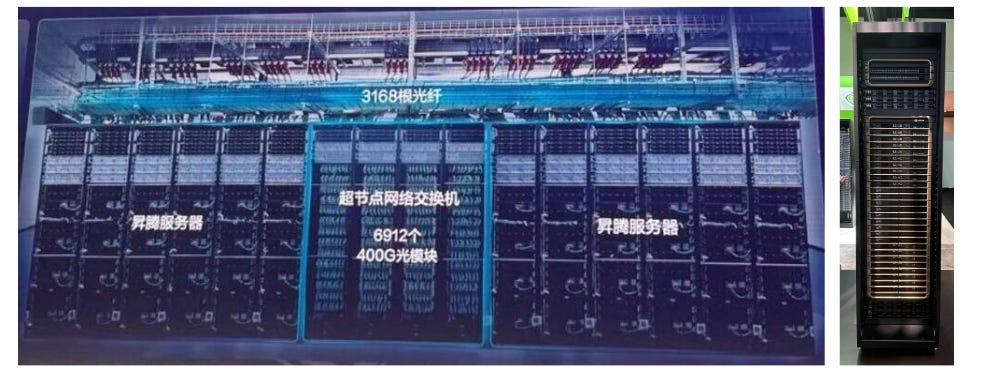
10x More AI Workers: The Power of U.S. Compute Dominance
This focus on preventing model parity misses the fundamental question: What are the true metrics of AI leadership? We reacted to DeepSeek because it narrowed the gap between U.S. and Chinese model capabilities as measured by benchmark leaderboards and model rankings. However, the fixation misses the broader technological competition.
For example, think of AI systems as “virtual employees” that can perform cognitive labor, replacing remote colleagues you’ve never met in person. The number of AI employees an economy can deploy hinges directly on its compute resources. With roughly 10 times more compute capacity than China, the U.S. can field proportionally more AI employees across its economy, in sectors as diverse as drug discovery, logistics optimization, industrial robotics, and AI research itself. This compute advantage therefore compounds into a broader economic advantage over time. As AI systems drive productivity growth and innovation, they strengthen the underlying factors of national power. While China may catch up in individual model quality for now, the true metric of AI leadership might lie in deploying and integrating these systems at scale. In that sense, America’s true moat isn’t just better models—it’s the capacity to deploy and integrate AI in the economy at scale.
Maintaining and Leveraging America's Compute Advantage
Both the Trump and Biden administrations deserve credit for recognizing early on that compute would be central to AI competition, taking key steps like restricting extreme ultraviolet (EUV) equipment exports to China in 2018 and banning AI chips in 2022—before ChatGPT captured public attention. But to stay ahead, the U.S. government must now build capacity to act quickly when reinforcing export controls while developing a clearer understanding of their actual impact. Rather than hoping to prevent temporary model parity, the U.S. strategy should leverage its substantial compute advantage to transform the broader economy and establish leadership in AI deployment worldwide. China may achieve competitive individual AI models this year, but this narrow benchmark gap is neither permanent nor strategically decisive. China will continue to tout breakthroughs to rattle U.S. confidence, but getting distracted and abandoning America's fundamental compute advantage would be a profound mistake.
While Silicon Valley take AI capex like an AGI moonshot, China's approach to innovation and emerging tech is much more methodical and long-term based. Some of the cultural and political differences actually do favor China.
More efficiency and innovation relative to less capital - more model optimization and innovation as a direct result of AI chip export controls and bans. Fairly predictable trajectories tbh.
Each year the argument for technological primacy gets more tenuous. The correct answer for a long time, is to increase the quality of the people. Instead the US system avoids this at all costs.
Long gone is any confidence that the neolib managers of the nation's wealth and power are interested in sharing its benefits. A common expectation in much of the West is these tools will be used as a more efficient way to exploit the people and enforce control.
The advent of AI actually is a vital subject, in my opinion. Seeing some of the latest advances, I'm starting to come around to the immense transformative potential of AI. But tech as a rule is readily duplicated, so chances are distant of AI plugging the geopolitical-power gap. I.e. the dream is fallacious, of "fixing" the slower US and G7 rate of development. That gap is fundamentally due to the West - and US especially - having first wasted and later atrophied much of its human capital.
But given the ability to combine parallel calculation in massive centralized servers, ability to reason, and naturally amazing powers of pattern matching, AI will nonetheless enable great transformations. It seems particularly well matched tool to create something akin to the "panopticon". That was once the dream of high modernism. Now rightly seen by most as dystopian, a "worst practices" pattern in the realm of thinking about how to structure society.
Question people might want to ask is, do we really want to have this technology emerge under a combination of (1) proto-fascist Trump administration (2) egged on by believers in the high-modernist panopticon vision of humanity such as Thiel or Musk - and (3) that techno vision paired with the boundless greed philosophy, exemplified by price gouging basic medicine like insulin, because hey, if your customer doesn't give you all their money they're free to die aren't they? Is that 3-way power combination really going to be the one to oversee deployment of AI in the US? When they fail to beat international rivals, they'll inevitably turn their tools inward. Probably sooner than later. Is that what we want in the next 3 years?