How China’s Education System is Driving AI Diffusion + Paid Reader Survey!
2,300+ new AI programs in universities: what is the deal?
We’re running our first ever subscriber survey! Responding will be hugely helpful to help chart out our coverage for 2024 and communicate with advertisers. The survey will take 3 minutes max. Five respondents will receive $100 and a one-year paid subscription for free. Thank so much for your help here.
ChinaTalk has extensively covered the importance of diffusion in a nation’s technological power, especially in the context of AI. Notably, Jeff Ding’s research found that, in contrast to the US’s decentralized and more diffusion-friendly ecosystem, “China’s diffusion capacity lags far behind its innovation capacity, … almost thirty places lower than its innovation-capacity ranking.”
Kashov Sharma, a soon-to-be UT Austin computer-engineering grad and policy research intern at the House’s Energy and Commerce Committee, reports on how China is planning to ramp up its diffusion capacity: massive investments in AI education to train a mid-tier, industrial-focused AI workforce.
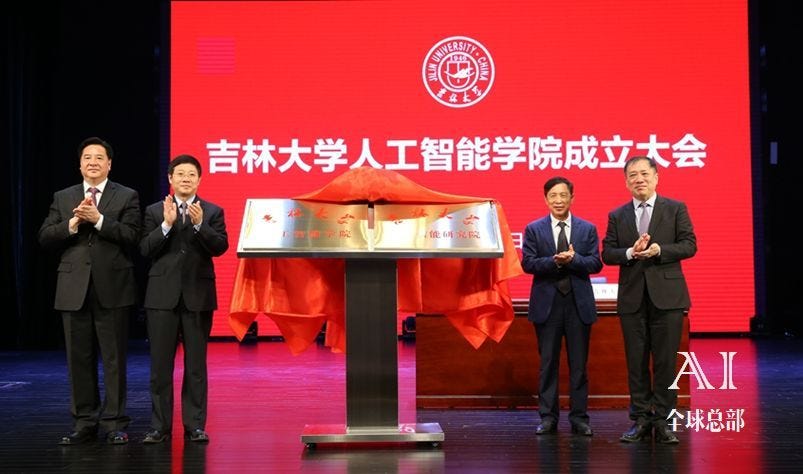
The US has lately enjoyed a steady stream of breakthroughs in large language models (LLMs) and diffusion models. These successes have fueled an explosion of US venture capital funding for generative AI startups focused on the tech and services sectors, like HarveyAI, Jasper, and Runway. That wave of optimism in the US contrasts with the relatively slower uptake of generative-AI startups in China. For instance, the much-hyped language model of Alibaba-backed startup 01.AI turned out to have been heavily based on Meta’s open-source Llama foundation model.
China’s apparent sluggishness in the generative-AI domain, however, is better explained by its strategic prioritization of industrial applications over applications in the service sector and traditional knowledge work. In particular, the CCP is banking on industrial-sector AI investments countering falling industrial productivity growth, hopefully launching it out of the middle-income trap.
To that end, Beijing has enlisted its Ministry of Education to promote cutting-edge machine-learning technology in its industrial sectors. As a result, Chinese universities have opened over 2,300 undergraduate AI programs, the majority of which are Applied AI programs focused on industrial applications.
This article discusses the MoE’s approach to AI higher education in two key respects:
First, how it aims to lower barriers for traditional industrial firms to adopt AI;
Second, how a large, mid-tier AI workforce stands to diffuse AI breakthroughs throughout China’s economy.
AI’s Business Model in Industrial Firms
The Ministry of Education’s unprecedented investment in applied-AI higher-education programs recognizes a fundamental problem that most industrial-focused AI firms face: building sector-specific AI solutions is incredibly time and capital-intensive and often cannot be achieved with a horizontal business model. That problem is endemic because — aside from niche industries like recycling (where successful Western startups like AMP Robotics have emerged) — most industrial sectors have company-specific data infrastructure. Manufacturing companies often run on software systems called Manufacturing Execution Systems (MES) and Supervisory Control and Data Acquisition (SCADA). These tend to combine in-house tools and a messy mix of software services built by legacy providers like SAP or Siemens.
Thus to build an AI model that can actually be deployed, these disparate systems must first be unified via a cross-system data pipeline. Much of this work is bespoke, involving the tedious process of building robust data pipelines between legacy, disconnected systems that often come from different providers with varying data schemas. Only after completing this arduous work can computer-vision models begin to be trained and deployed. And even after these complex data pipelines are built, training AI systems on industrial processes comes with its own challenges:
Given the multitude of products and processes used by industrial firms, each model must be trained on the specific systems of the company or product line. In other words, massive, industry-wide variety limits the scalability of industrial AI solutions.
Moreover, whenever a company changes a process or pivots to a new product, the firm must not only build out the new manufacturing process, but also retrain its computer-vision model — often a difficult feat if it’s a novel product or process without significant existing training data. As a consequence, without a robust dataset to tune machine-learning algorithms for the new process, manufacturers are often forced to manually test and produce physical training data until the model becomes reliable enough.
In the industrial AI space, the lucrative economics of a Software-as-a-Service (SaaS) company no longer apply due to the need for constant updates, limitations on scale across customers, and significant upfront costs. This challenge was demonstrated by Kai-fu Lee’s 李开复 AI industrial startup, AInnovation, which develops AI systems for industrial firms like the state-owned steelmaking group CISDI and construction giant China Railway No. 4. As AInnovation scaled its customer base, it was forced to increase software spending and deploy more proprietary, low-margin hardware, leading to gross margins crashing from 62.9% in 2018 to 29.1% in 2020.
Since industrial-sector AI can’t be adopted via a one-size-fits-all, horizontal solution, the industry demands a large, new, middle-tier of AI talent — more skilled than your average data analyst, but less costly than ML engineers and researchers. Beijing’s AI education strategy seeks to fill this exact niche of applied AI talent so that each industrial firm can hire in-house teams to build its own machine-learning infrastructure.
The fruits of this investment have begun to show. For example, companies like CATL are deploying computer vision at scale in their battery-manufacturing quality-control processes. That said, given the homogeneous, commoditized nature of batteries, it remains to be seen if Beijing can scale its AI efforts beyond such low-hanging fruit.
Education Diffusion for AI
Beyond facilitating the integration of existing AI technology into the industrial sector, Beijing’s AI higher-education policy also seeks to build the moderately educated workforce needed to diffuse emerging AI breakthroughs throughout the rest of the country. With many of the new applied AI programs located in rural and industrial regions, the new wave of AI programs seeks to spread AI’s benefits across China, rather than keeping them monopolized in service-focused coastal tech hubs like Beijing and Shenzhen.
Simultaneously, by focusing on this middle-tier AI talent, China stands to cost-effectively train workers who have the willingness to build out the data infrastructure its industrial firms need, while also understanding relevant breakthroughs on the cutting edge. Beijing achieves this by creating applied AI programs which omit teaching complex AI concepts, instead focusing on data models and the most basic machine-learning concepts — an approach which has proved to be quite scalable.
This approach differs from AI education in American universities. Speaking from personal experience (at UT Austin), American undergraduate AI courses rarely have students engage with and learn the messy but critical skills of building data pipelines and cleaning datasets, instead focusing on more theoretical topics like deep learning and transformers. Teaching such topics likely leads to more cutting-edge research breakthroughs, but isn’t as effective in cultivating a workforce capable of diffusing those breakthroughs to traditional industries across the broader economy.
In contrast, a graduate of Jilin University in Changchun 长春 — where the MoE started a new applied AI program — would be well-versed in building effective data pipelines for construction and manufacturing firms, while also being familiar with basic machine-learning concepts like convolutional neural networks (CNNs). And since these students are primarily learning how to build the infrastructure for AI systems, universities don’t depend on rare, top-tier AI professors — making applied AI programs easier to scale up.
With a large population of graduates with basic ML knowledge, Beijing doesn’t necessarily need to lead in AI breakthroughs. As new techniques emerge from China’s elite colleges like Tsinghua 清华 or Western labs like OpenAI, China’s moderately trained workforce can quickly learn and leverage these emerging techniques throughout the economy. For instance, although workers may primarily spend their time building data-cleaning processes and pipelines, they would still be comfortable enough with ML techniques to learn how to use CLIP or other image-processing breakthroughs, even if they are initially only familiar with basic techniques like CNNs. This workforce of domain-specialized, AI fast followers is particularly effective in the ML field, where knowledge is readily accessible through open-source papers.
Foreign observers would do well to remember that China’s focus on industrial applications of AI is consistent with the CCP’s vision for China to emulate the German model of high-end manufacturing, rather than the more services-oriented American economy. Indeed, the latest AI proposals coming out of Two Sessions — including the much-touted AI+ Initiative — were unsurprisingly geared toward converting “advancement in AI technology into tangible productivity in the real economy.”
Beijing’s mid-tier, diffusion-centered approach is also something America can learn from. Right now, US industrial-sector AI is applied mostly in high-end areas like semiconductor manufacturing equipment. But diffusion is at least as important as innovation — and it’s critical that the US leverages its own education infrastructure, including community and state colleges, to ensure that breakthroughs don’t stay bottled up in a few neighborhoods in Silicon Valley.
For more on this theme, our conversation with Jeff Ding was a classic:
A transcript here if you prefer:
GPTs and the Rise and Fall of Great Powers
Jeffrey Ding is a professor at George Washington University and the creator of the ChinAI Substack. He argues in a recent paper that great powers must harness general-purpose technologies if they want to achieve global dominance. In this show, we discuss the historical underpinnings of that argument and apply it to AI today — drawing out policymaking lessons spanning centuries of technologically driven great power transitions. We also get into:
And if you missed it, make sure to read up on the AI proposals coming out of the highest levels of the Chinese government:
Two Sessions on AI: Strategy, Security, and Sovereignty
Nancy Yu delivers the most comprehensive coverage to date of all the important AI proposals coming out of Beijing — Li Qiang’s “AI+ Initiative,” “sovereign AI,” “new quality productive forces” and AI, the pursuit (and dangers) of AGI, AI security, and generative AI erasing Chinese culture.
Some good points in this piece, but I think it conflates diffusion of AI throughout the economy (which is desirable for growth) and integrating AI into physical production processes (which China's AI education is focusing on). As the Macro Polo article (https://macropolo.org/chinese-university-ai-ambitions/) cited in the piece notes, China faces challenges integrating AI into industrial processes (e.g. due to risk aversion among Chinese enterprises) and there is a mismatch between AI education and industry demand for AI.
Services currently account for 55% of Chinese GDP and experts say it needs to increase that percentage further to reduce problems caused by excess manufacturing capacity, so it doesn't seem sensible for applied AI courses to be so weighted towards physical production processes.
The U.S. approach is not distributed it is totally elitist. AI for the 1%. China can use or steal the U.S. leading edge research and focus on applications. Smart. And will help millions not just an elite group in Silicon Valley.